In the fast-paced world of artificial intelligence, where yesterday’s breakthroughs quickly become today’s basics, Infrrd has done what others only dream of—cracked the code to making AI teach itself. We’re not just pushing boundaries; we’re erasing them entirely with our revolutionary approach towards Prompt Tuning.
Forget the hype and empty promises—LLMs have long been plagued by inconsistency and inaccuracies, often spitting out responses that sound convincing but fall flat on facts. It’s a phenomenon less widely known as “hallucination,” and it’s been a thorn in the side of AI developers everywhere. But that’s where Infrrd steps in—leading the charge with a game-changing solution that redefines how LLMs learn, adapt, and deliver results. Before getting deeper, let’s clear the basics first.
What is Prompt Tuning?
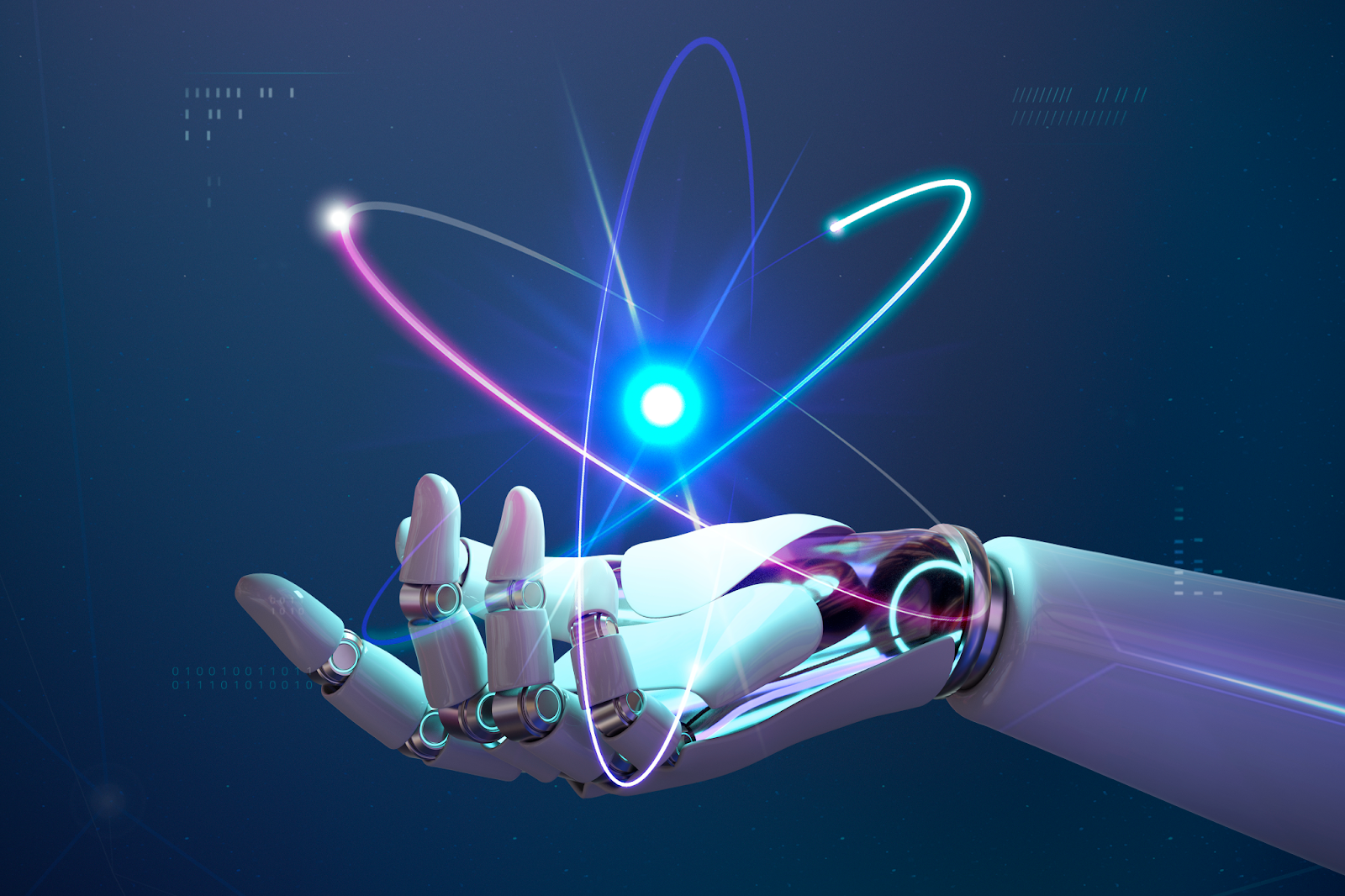
Imagine tuning a musical instrument to get the perfect pitch—well, Prompt Tuning works the same way for AI. Instead of retraining an entire language model from scratch (which is like trying to rebuild an engine), it fine-tunes the "prompts" (the questions or instructions we give the AI) to ensure it delivers the most accurate, relevant answers. Think of it as crafting the perfect question to unlock the most precise response from the AI—making it smarter, faster, and far more reliable. It's a game-changing shortcut that turns ordinary AI into a finely-tuned powerhouse without the hefty cost of starting from zero!
The idea behind Prompt Tuning is simple: optimize the way prompts (the instructions or queries) are crafted so that the LLM produces the most precise, relevant, and accurate output. This method can significantly reduce the notorious “hallucination” issue where models generate convincing-sounding but incorrect answers.
In essence, Prompt Tuning allows us to achieve more with less, improving accuracy without the need for extensive retraining or massive data sets. This makes it an incredibly important breakthrough for applications where precision is critical.
But Why Does Prompt Tuning Matter?
Prompt Tuning is a breakthrough in AI because it addresses several core challenges in machine learning and natural language processing:

- Improved Accuracy: By optimizing the input prompts, models become more accurate and contextually aware, reducing errors such as hallucinations.
- Cost-Effective: Unlike full-scale model retraining, Prompt Tuning is far less resource-intensive, requiring only minor adjustments to the input rather than large datasets or powerful computing infrastructure.
- Scalability: This technique makes it possible to implement AI solutions across various industries without the need for custom-built models, which are often time-consuming and costly to develop.
- Time-Efficiency: It allows businesses to quickly deploy AI applications with improved accuracy and consistency, cutting down the time spent on manual interventions.
How Prompt Tuning Rewrites the Rules
Prompt Tuning isn’t about endlessly retraining massive models. It’s about fine-tuning how prompts are structured to get the best possible responses from pre-trained LLMs. Instead of overhauling entire architectures, we zero in on optimizing inputs—making the models more precise and less prone to those infamous hallucinations.
There are two primary types of Prompt Tuning:
- Hard Prompt Tuning: Manually crafting and refining prompts using domain expertise and specific task requirements.
- Soft Prompt Tuning: Employing trainable embeddings optimized through backpropagation, allowing the model to discover the most effective prompts without altering its core structure.
By leveraging Prompt Tuning, we minimize reliance on massive datasets and eliminate the constant need for human tweaking. It’s a dynamic and adaptable method that transforms how LLMs perform, making them more reliable for specialized applications.
The Real Challenge in Prompt Tuning — LLM Accuracy
Large Language Models (LLMs) have revolutionized countless industries, automating tasks that require language understanding and generation. But despite their undeniable potential, they come with a glaring issue—the tendency to produce inaccurate, misleading, or downright nonsensical outputs. This inconsistency becomes especially problematic when dealing with complex, semi-structured, or unstructured documents, where even minor errors can snowball into significant risks.
Traditional methods to tackle these inaccuracies often involve extensive human intervention—meticulous prompt engineering, endless manual adjustments, and continuous oversight. While this hands-on approach can improve accuracy to some extent, it’s far from scalable and definitely not sustainable. The industry has been crying out for a smarter, more autonomous solution. Enter Infrrd’s revolutionary Prompt Tuning approach.
Infrrd’s Groundbreaking Multi-Agentic Framework
While most are still grappling with basic prompt adjustments, Infrrd has taken it a step further. Our Multi-Agentic Framework leverages Prompt Tuning to create a self-learning, self-improving AI ecosystem. This approach employs multiple LLMs that continuously interact and learn from each other, drastically reducing the need for human intervention.
Key Innovations in Infrrd’s Framework:
- Task-Specialized Agents: Tailored agents handle specific tasks based on complexity, from simple operations to advanced reasoning and coding tasks.
- Automated Rule Optimization: Dynamically identifies the best-fit rules by utilizing multiple LLMs to adapt to varying data structures.
- Entity-Type Handling: Accurately distinguishes between similar entities, reducing false matches and enhancing precision.
- Overfitting Mitigation: Ensures the model doesn’t overfit by accurately distinguishing between true negatives and false positives.
- Continuous Feedback Loop: The system self-adjusts without human intervention, maintaining long-term accuracy and consistency.
Real World Impact
We put this innovative framework to the test with some of the biggest names in the industry. The results were nothing short of impressive—accuracy rates soared as the system refined itself through continuous learning. However, we did encounter some challenges:
- Ground Truth Not Present in Context: Sometimes, data was presented differently (e.g., Pounds vs. LBS), making accurate extraction challenging.
- Low Occurrence of the Entity: Fields with mostly empty data (>90%) hindered effective rule formation.
- Limited Data Availability: Running rule optimization with insufficient data didn’t guarantee robust accuracy.
Despite these minor setbacks, areas where accuracy improved have been marked for further evaluation and fine-tuning—demonstrating the system’s ongoing capacity for learning and adaptation.
Why Prompt Tuning is a Game-Changer for AI: Uncover the Surprising Truths
1. Tackling Hallucinations for Reliable AI Outputs
One of the biggest challenges facing Large Language Models (LLMs) is the issue of hallucinations—when AI generates seemingly accurate but factually incorrect information. Enter Prompt Tuning, which has proven to dramatically reduce these hallucinations, making AI responses more factual and trustworthy. A study from Stanford's AI Lab demonstrated that this technique significantly minimizes errors, transforming the reliability of AI-generated content.
2. From Rigid Rules to Dynamic Learning:
Gone are the days of painstakingly crafting static prompts. Prompt Tuning is pushing AI to evolve beyond rigid, rule-based approaches by enabling it to "learn" how to structure inputs more efficiently. Research has highlighted how this adaptive learning model beats traditional prompt engineering, creating smarter AI that continuously improves.
3. Saving Resources, Boosting Performance:
Why retrain an entire model when you can fine-tune existing ones? Prompt Tuning is a breakthrough in resource optimization, allowing AI models to improve performance by up to 30% without the need for additional data training. Researchers found that this approach drastically cuts down on computational costs, streamlining the process for developers and businesses alike.
4. Unlocking Self-Improving AI Systems:
Imagine AI that not only improves over time but does so autonomously, with minimal human oversight. That’s the power of Prompt Tuning. As AI refines its prompt understanding, it becomes increasingly effective at processing complex queries.
5. Transforming Industries with Precision and Speed:
Prompt Tuning’s impact goes beyond basic tasks—it's redefining how industries like healthcare, finance, and legal sectors handle critical applications. For example, in finance, initial trials of Prompt Tuning saw a 25% reduction in loan processing time, thanks to its ability to generate highly accurate, tailored prompts. This means faster, more reliable decision-making across sectors.
So, What’s Next in Prompt Tuning?
Prompt Tuning represents a major leap forward in AI technology, offering a solution to many of the current limitations of LLMs. From reducing inaccuracies and improving efficiency to creating self-improving systems, this technique is shaping the future of artificial intelligence. Infrrd’s innovation in this area is setting a new benchmark, and as we continue to develop these technologies, the possibilities for what AI can achieve are endless.
The best part? Infrrd is already leading the charge in turning the concept of AI teaching itself into a reality. With Prompt Tuning, we are not just improving how AI works; we are unlocking new dimensions of efficiency, accuracy, and scalability that will transform industries.
Experience the future of autonomous AI with Infrrd—where machines teach themselves and accuracy is never compromised.
FAQs
Using AI for pre-fund QC audits offers the advantage of quickly verifying that loans meet all regulatory and internal guidelines without any errors. AI enhances accuracy, reduces the risk of errors or fraud, reduces the audit time by half, and streamlines the review process, ensuring compliance before disbursing funds.
Choose software that offers advanced automation technology for efficient audits, strong compliance features, customizable audit trails, and real-time reporting. Ensure it integrates well with your existing systems and offers scalability, reliable customer support, and positive user reviews.
Audit Quality Control (QC) is crucial for mortgage companies to ensure regulatory compliance, reduce risks, and maintain investor confidence. It helps identify and correct errors, fraud, or discrepancies, preventing legal issues and defaults. QC also boosts operational efficiency by uncovering inefficiencies and enhancing overall loan quality.
Mortgage review/audit QC software is a collective term for tools designed to automate and streamline the process of evaluating loans. It helps financial institutions assess the quality, compliance, and risk of loans by analyzing loan data, documents, and borrower information. This software ensures that loans meet regulatory standards, reduces the risk of errors, and speeds up the review process, making it more efficient and accurate.
Yes, AI can identify and extract changes in revised engineering drawings, tracking modifications to ensure accurate updates across all documentation.
Yes, advanced AI tools can recognize and extract handwritten annotations from engineering drawings, capturing important notes and revisions for further processing.