How to Choose the Best AI for Image Processing - A Quick Start Guide
Imagine how much more valuable your data would be to your business if your document-intake solution could extract data from images as seamlessly as it does from the text.
Thanks to deep learning, intelligent document processing (IDP) is able to make use of AI for image processing to not only automatically classify photos, but also describe the various elements in pictures and write short sentences describing each segment with proper English grammar.
Inteligent Document Processing leverages a deep learning network known as CNN (Convolutional Neural Networks) to learn patterns that naturally occur in photos. Intelligent Document Processing is then able to adapt as new data is processed, using Imagenet, one of the biggest databases of labeled images, which has been instrumental in advancing computer vision and AI image processing.
One of the ways this type of technology is implemented with impact is in the document-heavy insurance industry. Claims processing starts with a small army of humans manually entering data from forms.
Image Processing Use Cases
The use cases of AI-powered image processing are rapidly evolving across industries.
For example, in real estate, AI for image processing is revolutionizing how properties are evaluated. From identifying property types, lot sizes, and amenities through satellite and listing images, to flagging compliance risks in scanned floor plans, AI-powered systems reduce manual review time and boost decision-making accuracy. With Intelligent Document Processing (IDP), real estate firms can even auto-classify documents like property deeds, inspection reports, and lease agreements, turning scanned paperwork into structured, searchable data.
In the financial sector, where document volume is high and visual data comes in all forms, from bank statements to scanned contracts, AI for image processing dramatically speeds up workflows. These image processing tools, combined with Intelligent Document Processing capabilities, allow systems to identify fields like signatures, watermarks, tables, and logos with precision. This is crucial when verifying borrower identities, detecting fraud, or comparing financial statements during audits.
Image Processing in Intelligent Document Processing
Most impressively, Intelligent Document Processing doesn't just extract data, it understands context. Whether it’s reading handwritten annotations on an insurance claim form or pulling loan terms from a photographed Closing Disclosure, it delivers structured, reliable outputs even from low-quality image files. That means no more wasting time on data re-entry or error-prone OCR workarounds.
In a typical use case, the claim includes a set of documents such as: claim forms, police reports, accident scene and vehicle damage pictures, vehicle operator driver's license, insurance copy, bills, invoices, and receipts. Documents like these aren’t standard, and the business systems that automate most of the claims processing can’t function without data from the forms.
To turn those documents into data, the Convolutional Neural Networks are trained using GPU-accelerated deep learning frameworks such as Caffe2, Chainer, Microsoft Cognitive Toolkit, MXNet, PaddlePaddle, Pytorch, TensorFlow, and inference optimizers such as TensorRT.
.webp)
Neural networks were first used in 2009 for speech recognition, and were only implemented by Google in 2012. Deep learning, also called neural networks, is a subset of machine learning that uses a model of computing that's very much inspired by the structure of the brain.
"Deep learning is already working in Google search and in image search; it allows you to image-search a term like 'hug.' It's used to getting you Smart Replies to your Gmail. It's in speech and vision. It will soon be used in machine translation, I believe." said Geoffrey Hinton, considered the Godfather of neural networks.
Deep Learning models, with their multi-level structures, as shown above, are very helpful in extracting complicated information from input images. Convolutional neural networks are also able to drastically reduce computation time by taking advantage of GPU for computation, which many networks fail to utilize.
Let’s take a deeper dive into Intelligent Document Processing model's image data preparation using deep learning. Preparing images for further analysis is needed to offer better local and global feature detection, which is how Intelligent Document Processing enables straight-through processing and drives ROI for your business. Below are the steps:
Step One in Image Processing: Image Classification
For increased accuracy, image classification using CNN is most effective. First and foremost, your Intelligent Document processing solution will need a set of images. In this case, images of beauty and pharmacy products are used as the initial training data set. The most common image data input parameters are the number of images, image dimensions, number of channels, and number of levels per pixel.
With classification, you are able to categorize images (in this case, as beauty and pharmacy). Each category again has different classes of objects as shown in the picture below:
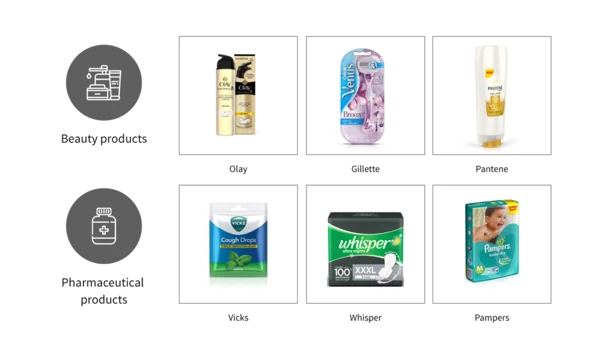
Step One in Image Processing: Data Labeling
It’s better to manually label the input data so that the deep learning algorithm can eventually learn to make the predictions on its own. Some off the shelf manual data labeling tools are given here. The objective at this point will be mainly to identify the actual object or text in a particular image, demarcating whether the word or object is oriented improperly, and identifying whether the script (if present) is in English or other languages.
To automate the tagging and annotation of images, NLP pipelines can be applied. ReLU (rectified linear unit) is then used for the non-linear activation functions, as they perform better and decrease training time.

To increase the training dataset, we can also try data augmentation by emulating the existing images and transforming them. We could transform the available images by making them smaller, blowing them up, cropping elements etc.
Step One in Image Processing: Using RCNN
With the usage of Region-based Convolutional Neural Network (aka RCNN), locations of objects in an image can be detected with ease. Within just 3 years the RCNN has moved from Fast RCNN, Faster RCNN to Mask RCNN, making tremendous progress towards human-level cognition of images. Below is an example of the final output of the image recognition model where it was trained by deep learning CNN to identify categories and products in images.
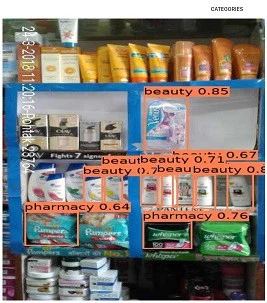
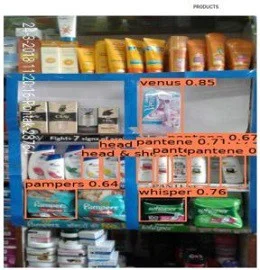
If you are new to deep learning methods and don’t want to train your own model, you could have a look on Google Cloud Vision. It works pretty well for general cases. If you are looking for a specific Intelligent Document Processing solution or customization, our ML experts will ensure your time and resources are well spent in partnering with us.
Chat with us at www.infrrd.ai or schedule a demo to learn more about how Intelligent Document Processing can drive business value from your data.
FAQ On Deep Learning Image Processing
Which algorithm is best for AI in image processing?
The best algorithm depends on the specific image processing task. For example, Convolutional Neural Networks (CNNs) are widely used in AI for image processing due to their accuracy in tasks like image classification, object detection, and segmentation. For document-centric tasks, Transformers and hybrid CNN-RNN architectures are also used, especially in Intelligent Document Processing solutions where layout and sequence understanding is critical.
Does AI-powered image processing have real-life applications?
Yes, absolutely. AI for image processing powers many real-world applications, such as automated medical imaging, facial recognition, manufacturing defect detection, and Intelligent Document Processing in industries like mortgage, insurance, and finance. For example, Intelligent Document Processing systems use AI to extract, classify, and validate data from scanned documents like invoices, closing disclosures, or engineering drawings.
How does an AI-based image processing model work?
AI-based image processing models use deep neural networks especially CNNs to detect patterns and features within images. These models process input images through multiple layers to identify and extract relevant data. In Intelligent Document Processing, this means understanding layout, reading handwriting, or identifying key fields like interest rates or claim amounts from complex forms.
What tools or programming languages are best for developing AI image processing models?
Popular tools include TensorFlow, Keras, and PyTorch all of which support building powerful models for image processing and Intelligent Document Processing. Python is the most widely used programming language for this work because of its flexibility and extensive libraries tailored for deep learning and computer vision.
Does AI for image processing require a lot of data?
Yes, effective AI for image processing models usually need large amounts of labeled image data to train accurately. This is especially true in Intelligent Document Processing, where the system must learn to handle different formats, layouts, and even low-quality scans. More data generally leads to higher accuracy and better generalization across document types.
Infrrd'a Image Processing for Engineering Drawings
Infrrd’s image extraction platform for engineering drawing leverages advanced AI, computer vision, and ML-driven Intelligent Document Processing to extract critical values from construction and manufacturing drawings with unmatched accuracy. This software for engineering drawings streamlines data extraction, ensuring faster and more precise image processing processing.
FAQs
IDP (Intelligent Document Processing) enhances audit QC by automatically extracting and analyzing data from loan files and documents, ensuring accuracy, compliance, and quality. It streamlines the review process, reduces errors, and ensures that all documentation meets regulatory standards and company policies, making audits more efficient and reliable.
Yes, IDP uses advanced image processing techniques to enhance low-quality documents, improving data extraction accuracy even in challenging conditions.
IDP efficiently processes both structured and unstructured data, enabling businesses to extract relevant information from various document types seamlessly.
IDP combines advanced AI algorithms with OCR to enhance accuracy, allowing for better understanding of document context and complex layouts.
IDP platforms can seamlessly integrate with ERP, CRM, and other enterprise systems, ensuring smooth data flow across departments.
IDP leverages AI-driven validation techniques to ensure that extracted data is accurate, reducing human errors and improving overall data quality.